Its many eyes have seen and recorded, measured and tested, and sent the data back to Earth. A single image can take an hour to send at 1k a second, and the data more than four hours to travel back to Earth. At the moment it is reserving its resources, concentrating on capturing and storing information. It will broadcast the high quality data, the full resolution images to the Deep Space Network’s eagerly listening giant parabolic antennas, when it has fewer tasks in the months ahead.
In the 1840s French mathematician Urbain Le Verrier used perturbations in the orbit of Uranus to predict the location of Neptune. The confirmation of Le Verrier’s calculations by German astronomer Johann Gottfried Galle led to the discovery of Neptune in 1846. Perturbations in the orbits of the giant outer planets lead astronomers to suspect that a 9th planet, “Planet X” existed. Its gravitational influence had been observed on the motions of Uranus and Neptune. American astronomer Percival Lowell obsessed with the discovery built observatories and calculated orbits in vain. 14 years after his death, on the 18th February 1930, using a telescope with a 13 inch objective lens with camera attached, specially built for the search in Lowell’s Flagstaff, Arizona observatory, Clyde Tombaugh discovered Planet X in the region predicted by Lowell by comparing a sequence of photographic plates. A tiny moving point of light against the background of the fixed stars in Gemini.
Lowell dreamed and calculated. Pluto was discovered in the region of space his mathematics predicted, and yet curiously enough, this proved to be a coincidence. Later observers confirmed Pluto was too small to have any gravitational impact on the outer giants, Neptune and Uranus. Was there then, a tenth planet? More accurate measurements from the Voyager 2 spacecraft showed that rather than a great outer planet, a miscalculation of Neptune’s mass accounted for the anomalies in Uranus’s orbit. That same data lead to a debate over Pluto’s classification as a planet, and it was reclassified by the International Astronomical Union as a dwarf planet.
Dreams and wonders, calculation and coincidence, measurement and observation had both created the 9th planet and uncreated it.
The initial photographs from the New Horizon’s flyby on July 14th have revealed Pluto to be a planet of stark contrasts and unexpected enigmas. Reddish, perhaps because of nitrogen in the dwarf planet’s atmosphere. There is a bright, vaguely heart shaped expanse, named Tombaugh, smooth from recent geological activity, perhaps flows and volcanoes of liquid carbon monoxide, within the last 100,000 years. A darker area, named Cthulhu (the dark alien god from the horror stories of H P Lovecraft), shows a rougher surface, with mountains at its edges comparable in height to those on Earth. More accurate measurements show it to be 2370km diameter, 1100km smaller than our Moon. There are ice caps at the poles, of frozen methane. Craters on the planetoid may be named after Star Trek characters; Kirk, Sulu, McCoy, Spock.
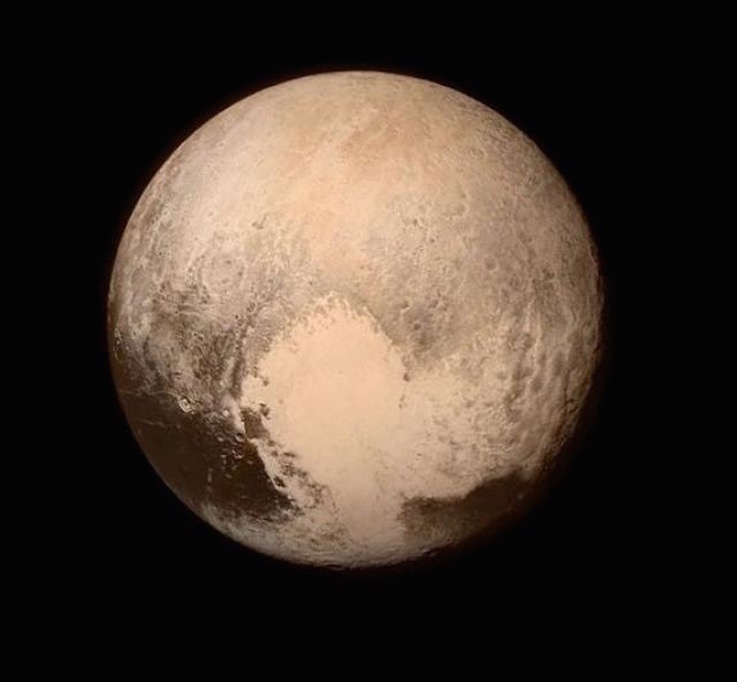
Despite the arrays of eyes, the dreams and imaginings, information returns at a trickle, and the terrain remains a mystery, a map, not a landscape, a cold Plutonic shore.
Only a few weeks before we visited the frozen Hades on the edge of our known space, Google took us deep into unexplored territory in a space as cold, as inhospitable, as alien, and as beautiful.
Neural networks are a way computers can emulate the processes of the brain. By feedback and a fractal-like repetition of patterns, of returning similarities and forgetting differences, neural networks remember and learn. Currently even our cleverest digital applications still need to be educated piecemeal. Voice recognition, face recognition, image recognition and search seem like miracles, but when we ask or search, the answers we receive are lists of probable matches from umpteen millions of stored possibilities. A search for cold Pluto could easily result in an icy planetoid, a frozen snack, a cartoon dog or a cthonic god. The thing, as yet does not learn.
Recent developments in the programming of neural nets have resulted in sudden advances in voice recognition and image classification. Instead of having to reference thousands of separate waveform pieces to recognize the correct word, the computer now has the potential to simply learn. The machine’s first efforts are confused, perhaps in the way a child that has not seen television before sees vague colours and shapes, some familiar, some not. But it is beginning to learn, how and why some neural net parameters are effective and others aren’t is still a mystery.
Google’s software engineers describe the process;
We train an artificial neural network by showing it millions of training examples and gradually adjusting the network parameters until it gives the classifications we want. The network typically consists of 10-30 stacked layers of artificial neurons. Each image is fed into the input layer, which then talks to the next layer, until eventually the “output” layer is reached. The network’s “answer” comes from this final output layer.
Fed millions of images of animals, then asked to identify an animal in a picture, different layers will go through different processes, finding corners, then edges, then shapes, then whole forms that have been stored as describing “animal”.
And yet in another test, asked to find a dumbell, the resulting image fails to distinguish between the weight lifting equipment, and the indistinct muscular figure lifting it. Or perhaps it already has a sense of humour?
Think of it this way. Imagine we feed a neural net images of of motorcars, bikes and motorbikes. If we then ask it motorcar – car + bike = the answer should = motorbike. If we ask it motorcar – two wheels + bike the answer should also = motorbike, but we could conceivably end up with Lady Penelope’s six wheeled Rolls Royce. It should be able to ignore what isn’t, but sometimes it may identify to excess what is.
A neural network, trained to identify animals, will find animal-like creatures in random shapes like clouds and even from images of like TV static, like the day dreams of children, or the terrors of madmen. The android learns, and dreams, and makes what it dreams, real.
To explore such questions Google released the code of their Inceptionist Deep Dream Neural Network, and like an idea in a neural net itself, it has proliferated, resulting in the pyschedelic, the fractal, the monstrous.
Here, we asked a Deep Dream net trained to identify animals, to find the inhabitants of the bare, cold, distant hellish realm of Pluto. It found for us something surprising but perhaps not wholly unexpected, crawling, on the planet’s face, something dark and of the underworld, scaled and tentacled, insectoid and hungry, something alien. The Pisces Luscus Chilopoda.
The Plutonian Fish-Eyed Centipede
Pluto was in the mythology of Greece and Rome the Lord of the Underworld and the Underworld itself. A taker and a keeper of human souls, the master of hidden things, jealously guarding the miserable dead and the earthly treasures in his subterranean realm. A guardian equally of nightmares and desires, in anthropomorphic and topographic forms the symbolic subconscious of the ancient mind.
The oracles of the ancients found truth in dreams, the fulfillment of which often bore dangerous fruit, Sigmund Freud saw a realm where our hidden terrors and everyday anxieties became narratives of the inexplicable, sublime and ridiculous and monstrous, Carl Jung saw a kind of interconnected sea in which dwelt our shared ideas, Phillip K Dick and saw a space negotiated between minds, not necessarily only human, where the divine interconnected and interconnected with the ordinary, where creation came out of awareness, and awareness out of creation, where androids dreamed not only of electric sheep, but of the soul, and in so doing, perhaps obtained their desire, and taught us of our own. William Gibson saw in that space between the cold hard structures of an immeasurable data space, in its errors, its flaws, its detritus, its unplanned interactions, in what we might consider the fraught dream of the computer, the anxiety of the aware machine, a virtuality that could hold universes.
From the Buddha we learn that with our thoughts, we create the world. What then do we create with our dreams, if not other worlds, illusory worlds, and the illusion of each other?
Does the myth create the monster, or the monster create the myth? And if the monster has now seen us, will it enter our dreams?